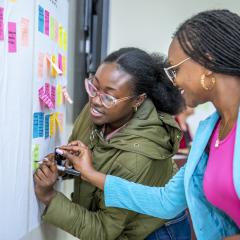
Towards a Model for Humanitarian UAV Coordination
February 6th, 2018
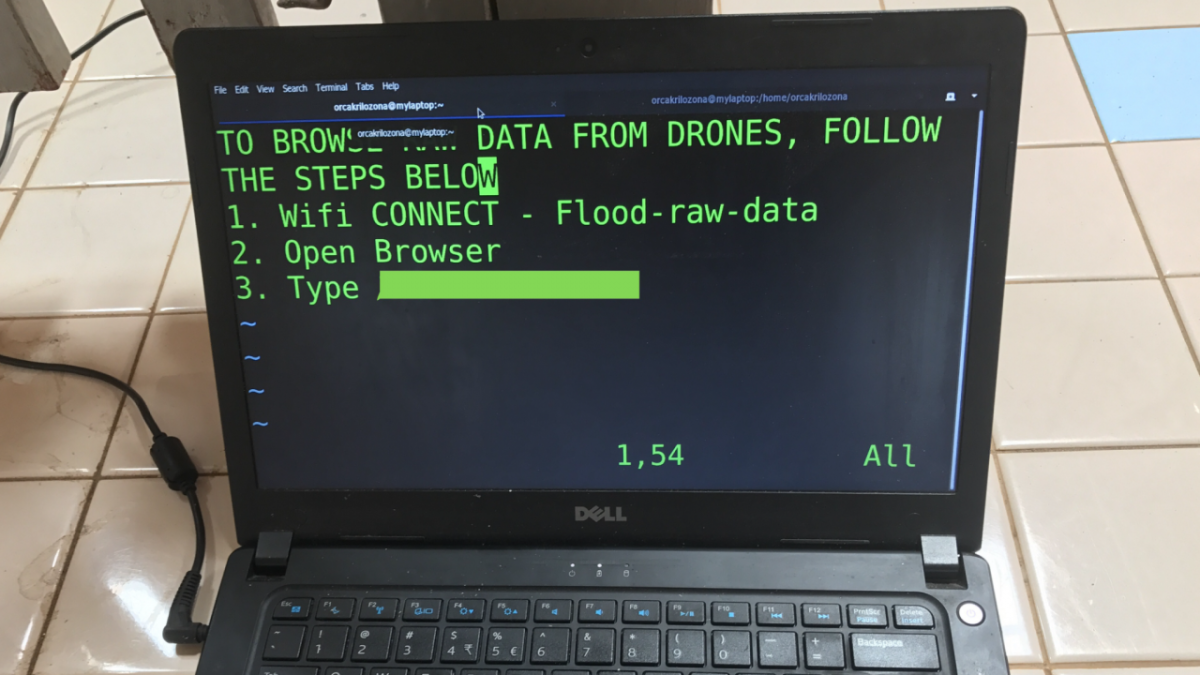
Authored by Joel Kaiser, Director of AidRobotics at WeRobotics
Over the course of 2017 my WeRobotics colleagues and I teamed up with multiple UN agencies and a handful of host governments to workshop and simulate the use of drones, or Unmanned Aerial Vehicles (UAVs), in humanitarian response. Several articles have been published about these events, which you can find at WeRobotics, Devex, WFP and UNICEF.
Each workshop included two full days of sessions that included case studies of previous UAV deployments, presentations from aviation, technology and humanitarian experts, and small group discussions for analysis and reflection. All of this culminated in a national capacity gap analysis that focused on preparedness and response challenges across three groups of participants: UAV Pilots, Data Analysts, and Response Coordinators. The third day involved a humanitarian response simulation during which the gap analysis was ground-truthed.
To identify the common challenges across all the workshops I performed a comparative analysis of the gaps from each workshop and used root cause analysis to perceive their underlying reasons. Then I modeled them within a high-level workflow of humanitarian UAV coordination to fully comprehend them within context. The result will be published in a forthcoming WeRobotics Discussion Paper on Humanitarian UAV Coordination and webinar on Tuesday, February 20th at 17h CET/11h EST. Please join the WeRobotics email-list for updates on both.
In this blog post I’ll focus on the three most prominent gaps: insufficient capacity in data analysis, lack of supportive regulatory frameworks, and a need for expert training to build cross-functional skills (and familiarity between Communities of Practice).
Gap 1: Insufficient Capacities in Data Analysis
The insufficient capacity by local actors to use aerial imagery in humanitarian response ranked as the most consistent constraint across all of the workshops. It manifests in nearly the entirety of the data value chain, from imagery processing to map reading, and should command the bulk of our attention and efforts.
This constraint finds its root cause in two domains, technological and analytical. Technological limitations prevent analysts from processing aerial imagery into orthomosaics for feature detection in a timely manner sufficient to the requirements of response coordinators. This constraint directly relates to the insufficient processing power of (even the most) high-powered laptops. Analytical limitations prevent the identification and extraction of decision-making information from aerial imagery in a timely manner. In some part, the constraints relate to each other.
Gap 2: Lack of a Supportive Regulatory Framework
No country visited had any special provision within their UAV regulations (where they existed) concerning the “validation” of pilots or vehicles, to say nothing of their deployment in humanitarian response. The result is that oft-mentioned deployment requirements, such as activation of pilot rosters or the tasking of registered UAVs, lacks policy guidance. This seems a crucial first-step since a roster of pilots requires some means of coordination, and a UAV registry will need to include certain parameters in order to support follow-on processes, such as the timely approval of flight plans.
A special provision for humanitarian response within national UAV regulations would also do well to reduce chaos when international actors are invited to respond and make attempts to import UAVs or parts to supplement deficiencies within an in-country fleet (granted, declaration of a system-wide level 3 emergency might solve this problem). The preparedness level in each country could be vastly improved through inclusion of provisions such as these within the regulatory policies governing UAVs.
Gap 3: Expertise training across different Communities of Practice
The need for training and certification of experts in piloting and data analysis was uniform across all countries. This comes as no surprise. However, if properly structured, expert training would not only help to strengthen national capacities in data analysis, but it would also serve as a means to prepare locally contextualized, yet globally interoperable, procedures and protocols.
The integration of UAVs into disaster management decision-making will trade upon trust. Building trust between CoPs requires experiential learning to tease out a shared understanding of the realistic capabilities within each CoP. Response coordinators don’t need to learn how to pilot UAVs or process imagery, but they need to know how those things happen. The same can be said for the other CoPs too. What we come away with is a need for both national centres of expertise as well as additional experiential learning events, such as hands-on training and simulations.
Integrated Gaps Analysis Framework
The framework below integrates all our findings from the multiple workshops carried out. The framework is also available here as a PDF.
The Compounding Effect of Bad Data
There is an oft-repeated data proverb: garbage in, garbage out. However, the humanitarian UAV coordination model below shows that the problem of ‘garbage in’ doesn’t simply end with ‘garbage out’. Instead, ‘garbage in’ results in a lot more work. Below we introduce a dynamic systems model to visualize the workflows that comprise humanitarian UAV coordination. This model is also available here as a PDF.
Blue arrows represent an increase, and red arrows represent a decrease. The ‘Situational Awareness gap’ results in the RC making an ‘Information Product request’ (thereby, gaps increase requests). The ‘Information Product request’ results in an ‘Imagery Collection tasking’ (requests increase tasks). On the far right side of the model the system ends with a red arrow because ‘Information Product dissemination’ results in a ‘New Situational Awareness’ (ie. the gap is resolved: information dissemination decreases gaps).
The focus of this model is on the two sub-systems, which are the circular feedback loops in the middle. In the first half we have the UAV Flight Operations sub-system: the big loop shows that UAV Flight Operations results in increased Aerial Imagery. Aerial Imagery that is fit for purpose results in a decreased number of UAV Flight Operations that are required. The small loop shows UAV Flight Operations results in decreased UAV Flight Readiness. UAV Flight Readiness experiences a delay before it can increase UAV Flight Operations (eg. UAV batteries take a long time to charge). This delay is unavoidable, even if we add more batteries. This provokes some questions: How is Aerial Imagery fit for purpose? What is the effect when is not/no longer fit for purpose?
Imagery Fitness
Aerial Imagery is fit for purpose when it satisfies the requirements of the intended analysis (and by extension, the analyst). It may be tempting to claim that very high-resolution imagery (eg. 2-3 cm) that contains more data for an analyst than low-resolution imagery, is more fit for purpose. However, this may not be true. Very high-resolution imagery comes at a very high cost, particularly in terms of longer flight times and longer processing times, both of which result in delays. What is the effect when imagery is not fit for purpose?
The answer is in the model just as it was experienced in the simulation: Unfit imagery requires re-collection (ie. another task and another flight operation), which decreases flight readiness. Hence, ‘garbage in’ creates an additional possibility than just ‘garbage out’: the work has to be re-done. While the UAV pilot re-flies the original operation to obtain fit imagery, other tasks continue to pile up. The compounding negative effect of unfit imagery needs careful attention since even just one poor dataset can severely foul the entire system.
How can imagery fitness be maximized? The answer is not found in the model, but we did gain some experience in the simulation. Imagery fitness relates to its range of usability for analysts. Imagery can also be unfit for a range of reasons, such as insufficient resolution or errors in geo-tagging. Imagery can also become unfit over time, which is a question of temporal resolution. Generally speaking, an orthomosaic with high spatial resolution is fit for many purposes, while video is fit for far less. However, orthomosaics require a significant time to acquire, compared to video which can be streamed in real-time. Some newly emerged tools can also increase the fitness of imagery, for example 360 drone imagery from Hangar, as well as a video mapping platform from Survae (pictured above).
The point here is that the model demonstrates that imagery fitness is where preparedness efforts are best applied, rather than trying to improve “UAV Flight Readiness” by adding more batteries or purchasing a bigger UAV with longer range. Greater research should be applied to acquiring and extending imagery fitness because this will have the most sustainable and durable pay off.
Imagery Tasking
In the second half of the model we find the Imagery Analysis sub-system. This sub-system behaves in much the same way as the one previous. For example, in the small loop, just like batteries in a UAV, analysts have a finite amount energy; with each Imagery Analysis performed the Analyst Capacity is consumed. After some rest they resume work. In the big loop, the result of their work is some sort of Information Product, which is either fit for purpose or not. “Unfit” Information Products put the system at greater risk than unfit Aerial Imagery because unfit Information Products may be the result of (ie. include) unfit imagery. Yet, how can an Information Product contain unfit imagery if the imagery has already been validated by the analyst? The answer to this is in the dark art of tasking.
Tasking is a complex topic that will be the subject of another paper. However, it is exactly where we should focus our preparedness efforts. Adding more analysts to solve the problem not only costs more money, but it also runs into the reality that there are very few analysts available (according to Gap 1). Our preparedness efforts must be put on ‘getting tasking right’. For this, we can learn a lot from other industries, such as healthcare.
Remember not so long ago when it was reported that patients were being asked to sign their body part prior to surgery? This could be called the mother-of-all-double-checks since the vast majority of wrong surgeries don’t occur because of a misdiagnosis, but rather mixups inside the operating room. In the same way, unfit Information Products aren’t produced because of misunderstanding of the Gap in Situational Awareness, but rather an error in expressing the gap into a task. Operating theaters move at a frenetic pace, just like a humanitarian response. And, just like surgeons, analysts are not always directly involved in the diagnosis of the problem.
The first thing that needs to be determined among other things involving the three groups involved, is who tasks and who is tasked? This ought to be studied and simulated because no matter how we how we design the system, the role of the Analyst is the most crucial to ‘get right’. And yet, globally, this CoP has the least capacity. We need to invest in analytical capacities in humanitarian response. But how?
Reinforce, Not Replace
WeRobotics perspective is that new processes and systems to guide the adoption of humanitarian UAVs will only find applicability and sustainability on the global scale if they are informed by operational actors and tested through real-world application on the local scale. To this end, we are committed to a bottom-up approach that localizes UAVs within national stakeholders, for iterative learning within localized emergencies. The principle of localization, that is to ‘reinforce, not replace’ in-country capacities and systems is the guiding principle behind three approaches to humanitarian UAV coordination preparedness favored by WeRobotics. These are: (a) Knowledge Transfer, (b) Real-time Mentorship, and (c) Contextualized Interoperability.
1. Knowledge Transfer not only involves specific trainings delivered by experts, but must also include a combination of deep-dive workshops and additional simulations targeted at insufficiencies at the local level.
2. Real-time Mentorship involves pairing of external experts, even remotely, with local actors engaged in a response in order to apply knowledge transfer to real-word scenarios during a response.
3. Contextualized Interoperability refers to the need to analyze and internalize local policies, cultures, and agendas within a globally interoperable (yet) local coordination model that is simultaneously beneficial for local actors and intuitive for international actors.
Given the rapid pace of technological change, neither outside experts nor well-supported locals will be able to succeed alone. Both will need to remain engaged in preparedness events that include focused discussion, idea consolidation, real-world application and impact evaluation. Finally, and most importantly, the results of these approaches, and others like them, must be shared widely and transparently, so that similar efforts may benefit from each others’ success.
Recent Articles
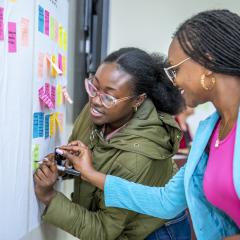
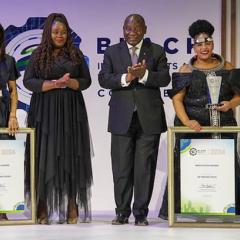